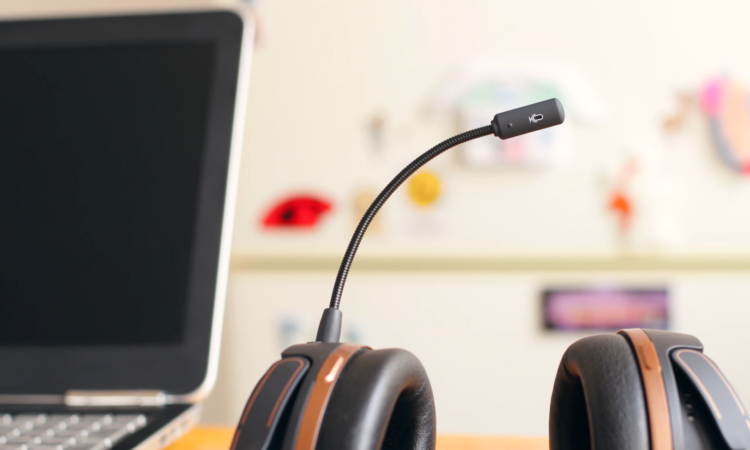
AHT (Average Handle Time) is one of the most relevant metrics in a call center, along with FCR, churn, or quality: a key indicator that acts as a compass, guiding you in the right direction to maintain a high level of service.
Respecting your customers’ time is the foundation of a good customer experience. But this premise competes with that of cost control and improving efficiency and productivity.
In other words, we find ourselves in a constant dilemma, trying to balance costs with a quality customer experience.
The question is: can we improve the customer experience while reducing call center costs? It’s one of the biggest challenges in the industry. But one way to approach this problem is by improving the AHT (Average Handle Time).
We will talk about it in depth, but we can already tell you that improving doesn’t always mean reducing, at least not reducing per se.
We will delve into all the details in this guide; from what AHT is and how to measure it, to key aspects to consider, including how artificial intelligence and machine learning have drastically improved the way to optimize this indicator.
What is AHT and how to measure it
AHT (Average Handle Time or Average Handling Time) is a KPI that helps us understand the average time of interactions with customers in a contact center.
This same indicator is also known as TMO, which stands for Average Operation Time in Spanish.
A common formula for measuring AHT is the following:
(Talk time + hold time + after-call time) / total number of calls
That is, AHT takes into account the amount of time an agent uses in a query or incident, both during the call and afterwards.
As you can see in the formula, the elements that affect AHT are self-explanatory, but when it comes to measuring and optimizing processes, starting with clear definitions is never a bad idea:
- Talk time: is the total time that your agents are talking to customers.
- Hold time: is the time the customer is on hold, i.e., the average time they wait until an agent attends to them. It is calculated by dividing the total wait time (the sum of all calls) by the number of calls received.
- After-call time (or ACW, After Call Work): is the time dedicated to tasks that the agent needs to carry out to resolve the query before being available for another call, such as sending emails, making notes, or updating comments in the CRM.
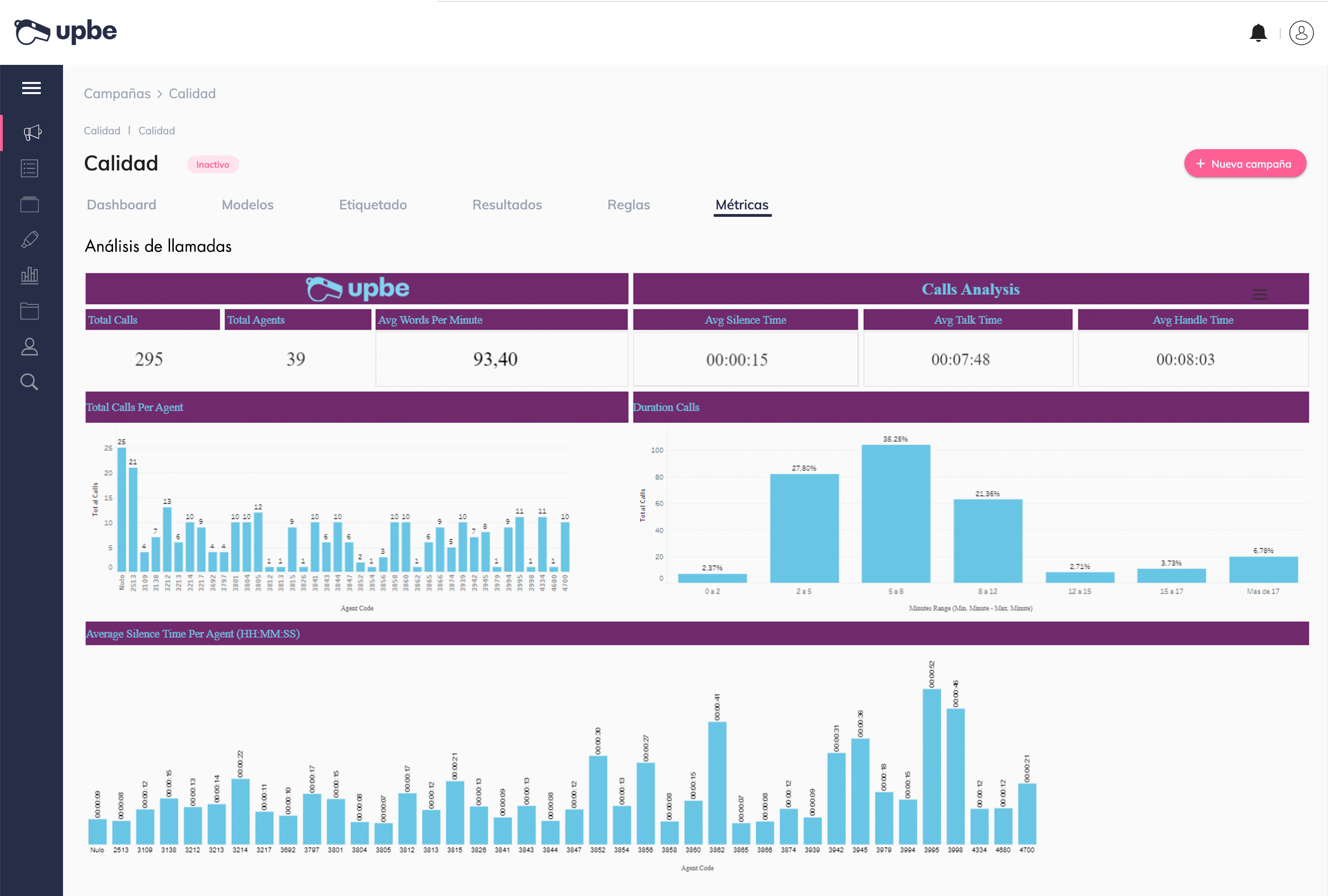
Both FCR (First Contact Resolution) and AHT (Average Handle Time) are metrics that speak to quality and efficiency in customer service operations in a contact center.
Interpreted correctly, these KPIs teach us a valuable lesson:
To be efficient and provide the best service, we must understand that each case requires time for its proper resolution and the best possible dedication from the agent, who must have the appropriate training and tools.
Key aspects before optimizing AHT
What is considered a good AHT? Is there a benchmark you should use to improve your AHT?
In this case, it depends much more on the industry or product. Although we advise the same as with FCR: understand it well, internalize the concept, define how to measure it properly, and establish ad-hoc follow-up KPIs for your business.
Don’t compare or try to follow market standards because it all depends on your culture (the importance you give to brand and customer experience), the industry you operate in, or the product or service you are supporting.
Although it’s not easy to share a standard AHT (Average Handle Time) by industry, there are important reflections to make before measuring this KPI or changing the way we do it.
These 4 questions are a good starting point to measure the average call time:
- Why do I consider reducing wait times vital?
- Is my call volume high? Do I need to improve compliance with other market standards such as the SLA (Service Level Agreement)?
- Will optimizing wait or conversation times help me receive more calls?
- How can I optimize the AHT?
As we mentioned earlier, reducing is not improving. In fact, reducing AHT is relatively easy, but it’s not a good practice by itself.
There are different lines of action, but first, you need to be clear that optimizing AHT means reducing call times while increasing (or at least maintaining) your levels of satisfaction.
Why is it important to internalize this? Because the level of analysis of your AHT cannot be biased.
All calls have their context, and to understand the AHT metric well, you need to adapt it to the circumstances.
For example, setting time limits for certain queries or incidents and pressuring your agents not to exceed them may reduce AHT. But in no case will it improve it if it happens at the expense of not giving the customer the necessary explanations, hurrying them, or even cutting off the conversation.
On the other hand, improving internal communication so that call transfers between agents are more fluid would reduce AHT while increasing customer satisfaction. In this case, we can clearly say that we have improved the metric.
In short, reducing AHT at all costs without understanding what’s at stake or risking customer satisfaction won’t get you very far. So, at this point, what specific actions can you take?
How to improve AHT (Average Handle Time)
Once you have understood the different aspects that come into play when measuring AHT, it’s time to take action.
Here, it is important that the hypotheses or proposed actions can be measured in isolation, starting with specific clusters of customers or highly segmented services or products.
These are the main ways to improve AHT:
- Understand the duration of agent responses, taking into account the appropriateness to the context of the call.
- Reduce unnecessary waits or silences throughout the service process, understanding the rhythm of the conversation and the meaning of the silences.
- Improve the flow of conversation patterns through the understanding of emotions and control of specific language expressions or concepts.
- Improve the training of your agents by developing continuous training programs that help them master all casuistics.
- Improve the logistics behind your calls, for example, optimizing redirects, or recording and analyzing calls to draw better conclusions.
- Monitor team performance and communication and operation flows to streamline interdepartmental workflows.
Improving AHT: Speech Analytics vs Artificial Intelligence
We already know that Speech Analytics technologies have helped contact centers to improve the interpretation and analysis of information in recent years.
This has led the sector to improve the quality and performance of customer service with more specific and efficient improvement proposals.
These are some of the AHT optimization actions that can be performed with standard Speech Analytics solutions:
- Identifying the reasons for calls, categorizing and specifying which processes can be improved.
- Analyzing how call times are distributed to detect ways to optimize them.
- Identifying agents with better satisfaction and AHT and scaling best practices to the rest of the teams.
- Comparing AHT within your teams and focusing effort on those departments that may need it the most (because their product or service is complex, for example).
But stopping there is only halfway, because context is everything in our sector. And with standard Speech Analytics tools, context is lost.
Furthermore, this type of work requires a lot of time for categorization and labeling. And to make matters worse, that effort will not serve you to optimize learning and create an optimized knowledge base.
Lastly, and by no means least, the analysis is based on a very small percentage of calls. Who would want to make vital business decisions based on 1% of calls when you can analyze 100%?
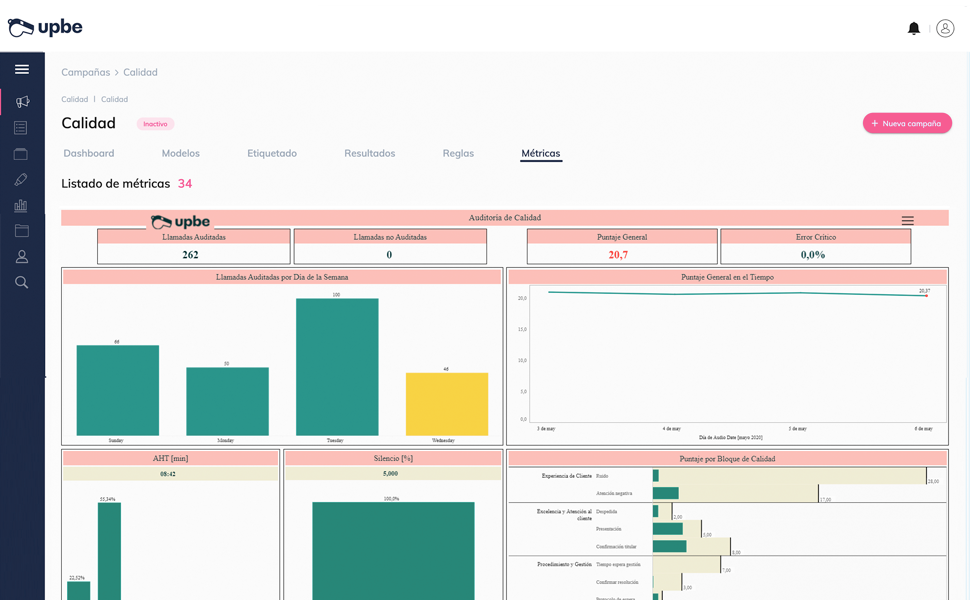
That’s why Upbe relies on artificial intelligence and machine learning to evolve contact center technology into what we call Conversational Intelligence.
How to Improve AHT with Conversational Intelligence
Understanding emotions and how they project in a conversation. The pauses or speed of conversations. The expressions or particular language concepts. Or the duration of agent responses.
All of these are key elements of customer satisfaction that we must respect and that Upbe identifies.
Let’s look at 6 specific ways Conversational Intelligence can help you optimize your Average Handle Time.
1. Optimize operation learning
This is achieved through machine learning. We focus on it through:
- A pre-configured quality template in Upbe that allows you to automate your quality process and detect inefficiencies and operational improvements.
- The platform’s customization area. You can train Upbe and adapt it to your context by creating specific items for your operation or particularities that you want to measure.
You train Upbe and Upbe will help you extract more specific learnings that you can apply to your operation based on your particular context.
What cases do I need to resolve faster and with less information? Where do I think my sales or service discourse provides too many details? In addition, the knowledge base, being based on the context of each call, will be much richer and you will have the opportunity to make it more powerful.
2. Improve agent performance
You will understand which agents solve queries better in more challenging environments: Which ones perform better with angry customers? Which ones have lower burnout and are more stable over time?
This information is critical not only for selecting the best agents for each case but also for scaling their knowledge to the rest of the team and for taking better care of them.
This will result in lower turnover, and keep in mind that talent retention greatly reduces the impact of a factor that directly affects AHT: the learning curve of new agents who join the call center.
3. Measure pauses efficiently
Speech Analytics allows you to measure pauses in the operation. If these occur in long calls, there may be bad practice by agents, and we must avoid that.
But standard Speech Analytics tools do not help you understand why these silences occur.
If you lose the context of the call, you may never identify the reason for their occurrence: Do they always occur at the same time? Always with agents of a certain experience? With the same product? What are the feelings before and after each silence?
4. Detect patterns and good practices
With Conversational Intelligence, your way of identifying conversational patterns and good practices evolves. Detecting these good practices and incorporating them into your knowledge base intelligently and automatically helps you scale them, improve training, and the onboarding process for new agents.
5. Reduce over-verification
This is a problem that can be solved with Speech Analytics. But not efficiently. Because that over-verification control that increases call time will depend on how you label it.
Another important point to consider is that Speech Analytics tags are literal. However, with Conversational Intelligence, the tool learns through examples and becomes more efficient at identifying these issues.
6. Understand emotions
Sentiment analysis, both from customers and agents, helps you make better decisions.
That information, the emotional context of a customer during the call, is a gold mine full of clues about possible improvements or changes in the sales pitch.
If you were told that a type of incident generates dissatisfaction in a high percentage of cases thanks to sentiment analysis, wouldn’t you change your way of managing those queries?
Still not clear on how Artificial Intelligence can help you in your Call Center? Request a demo, and we will explain in detail how we can help you improve both AHT and other performance indicators.
4 Comments
Comments are closed.