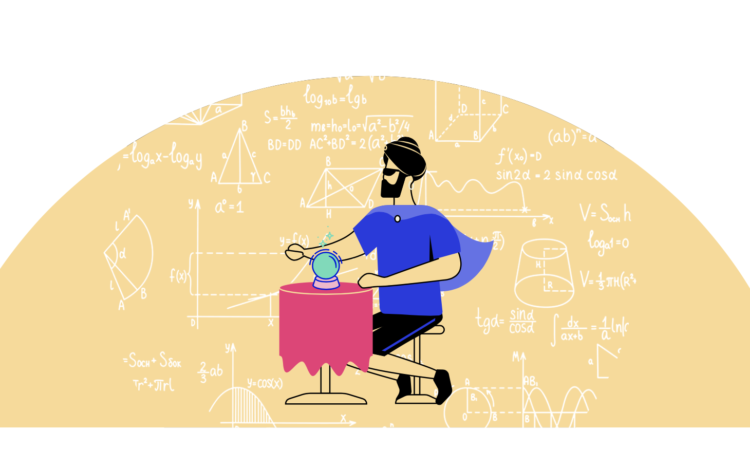
In the series of articles we are sharing about the technology that supports Upbe, we have already talked about fundamental issues. Such as the differences between Artificial Intelligence, Machine Learning, and Deep Learning, or the differences between NLP, NLU, and NLG. Today we will talk about ASR.
In the previous article, we talked about the potential of these techniques in contact centers, detailing the environments of tools and techniques that accompany NLP. In these environments, Speech Technology systems are located, which are two:
- Speech recognition systems (ASR)
- Text-To-Speech synthesis systems
These systems act as an interface between people and NLP systems. These technologies are the channel that allows communication between humans and machines. In the case of ASR, it allows communication between a human sender and a machine receiver, allowing NLP modules to receive a transcription of text. And Text-To-Speech systems establish communication where the machine is the sender and the human is the receiver by converting text into spoken speech.
Today, we are going to delve into Speech Recognition Systems (ASR – Automatic Speech Recognition). These systems are fully integrated into our daily lives. As technology, it is already validated since they are systems that voice assistants (Apple, Google, or Amazon) or messaging applications (for dictation, for example) rely on.
What is ASR?
In short: the audio that enters is converted into text. To convert it, in between, the audio has to be converted into a file that can be read by the machine. This means that the tool works with acoustic and language models.
Acoustic models contain a statistical representation of a sound or phoneme. It is created using many acoustic data. The language model statistically represents the probability in which words could occur or happen. That is, these models estimate the probability of certain phonemes appearing that mean certain words.
The goal of the acoustic model is to create a set of probabilities that represent all the language sounds that need to be recognized. To create acoustic models, you have to determine which sounds you want to represent or which probabilistic model you will use.
Those models determine the relationship between audio signals and language phonemes. Meanwhile, the model concludes which sounds fit with which words and phrases.
In steps:
- You speak to a software.
- The device creates text files.
- The file is cleaned from noise by the software.
- The file is divided into phonemes.
- The ASR system, based on probability from the language model, combines the phonemes and transcribes the original audio into text.
Now that it understands, the ASR system can respond by generating a transcription, understanding your context and responding sensibly. That is fundamental, and more applied to the analysis environment in which a large company or contact center operates. Converting unstructured data into structured information to analyze is differential for business.
In this sense, it is also important to highlight that an ASR system is capable of interpreting jargon, particular language usage, or accents with the appropriate technology suite. This is an approach that we are currently working on at Upbe because we know that there is a lot of business intelligence in properly interpreting this information.
What applications do Speech Recognition Systems have?
The applications of ASR (Automatic Speech Recognition) systems are very diverse. As we said at the beginning, it is a technology that is fully integrated into our daily lives. Here are several examples:
- Telephony: dictation systems, personal interface activation, message transcriptions, voice searches, or automatic translations are all common based on Speech Recognition Systems.
- Automotive: any voice instruction that a car can understand and manage, such as making calls, turning on the radio, or even opening a specific application.
- Home automation: all kinds of hardware that receive instructions and react to specific commands. This includes both Alexa and Google Home. Or any command to turn lights on or off or regulate the thermostat.
- Military applications: to have autonomy and independence during flight, there is a lot of technology based on ASR systems to change transmission frequencies, initiate auto-flight modes, or deploy parameters to establish flight coordinates.
- Audiovisual: it is common to use Speech Recognition technology to subtitle programs, both live and on-demand.
- Legal field: there are very interesting initiatives to optimize the transcription of information that is so necessary in the sector or for file searching.
- Call Center: focused on customer voice analysis, automation of quality and compliance controls, or improving effectiveness in sales campaigns.
And much more, such as IVR systems, robotics, applications in the video game industry, automatic translations, etc.
How can ASR improve call efficiency?
Looking at the previous list, which can be expanded further, of the applications of ASR systems, their relevance is understood. All this information, which we can pass from audio to text, is very common and complex. So much so that its applications, in the case of the contact center, mean completely revolutionizing an industry or sector.
Transcribing a dictated audio seems simple, but this generally does not happen in your call center calls. There are many interferences that ASR systems are capable of separating and analyzing. There are contexts of great complexity, with highly compressed audio recordings, voice overlaps, or background noise that distort what is in the audio.
In addition, generally, the speakers speak at different speeds, with diverse emotions, and even accents or jargon. This is what makes the process complex and requires the technology at its disposal.
How do we know if the ASR system works?
There are two metrics to evaluate if our system works properly:
- Word error rate, which measures the percentage of incorrect characters. It does this by analyzing the number of deleted, substituted, or inserted words that we have to intervene to get the real transcribed phrase.
- Sentence error rate, which measures the percentage of intervened sentences in a text.
Generally, the most valid or used is WER (word error rate). How is it calculated? To calculate the WER, we have to calculate the number of substituted, inserted, or deleted words between the correct version of the text and the version that comes out of the ASR system as it is. In this case, we have tested the dictation functionality of a standard mobile phone brand:
In an example where the words highlighted in yellow are wrong (and the yellow ones are the correct option), the WER is 9.4%. We understood that there are 5 modifications out of a total of 53 words.
Examples of successful implementation of ASR
Automatic Speech Recognition (ASR) has been a key tool for Contact Center as a Service (CCaaS) companies in their quest to automate and improve customer query processing. By using ASR solutions, companies can offer more flexible and satisfactory customer service, and have access to advanced technologies and analytics based on industry best practices.
Although old speech recognition technology was inaccurate due to industry-specific jargon and poor call quality, end-to-end deep learning has enabled the creation of accurate models with new data. ASR solutions are divided into two categories: speech recognition and speech comprehension. Both are particularly relevant to the call center, as it helps improve voice recognition and understanding the meaning behind what is being said.
Voice analytics can be implemented in a call center to automate the following functions:
- Quality verification
- Content moderation
- Trigger word identification
- Enabling self-service
- Etc.
However, implementing voice analytics in a call center also presents challenges, such as accurate and cost-effective transcription of conversations, generating meaningful insights from transcribed voice data, and effectively applying insights to improve final outcomes. To overcome these challenges, companies must invest in accurate and predictive analytics tools and find suitable partners to execute these initiatives successfully.
Despite these challenges, the advancement of deep learning has made it possible to transcribe voice to text with high accuracy at the cloud level, making this technology more accessible for companies, allowing voice analytics to provide valuable insights for businesses and improve customer satisfaction in the call center.
2 Comments
Comments are closed.